Amazon SageMaker Model Deployment
Easily deploy and manage machine learning (ML) models for inference
Amazon SageMaker makes it easy to deploy ML models to make predictions (also known as inference) at the best price-performance for any use case. It provides a broad selection of ML infrastructure and model deployment options to help meet all your ML inference needs. It is a fully managed service and integrates with MLOps tools, so you can scale your model deployment, reduce inference costs, manage models more effectively in production, and reduce operational burden.
How it works
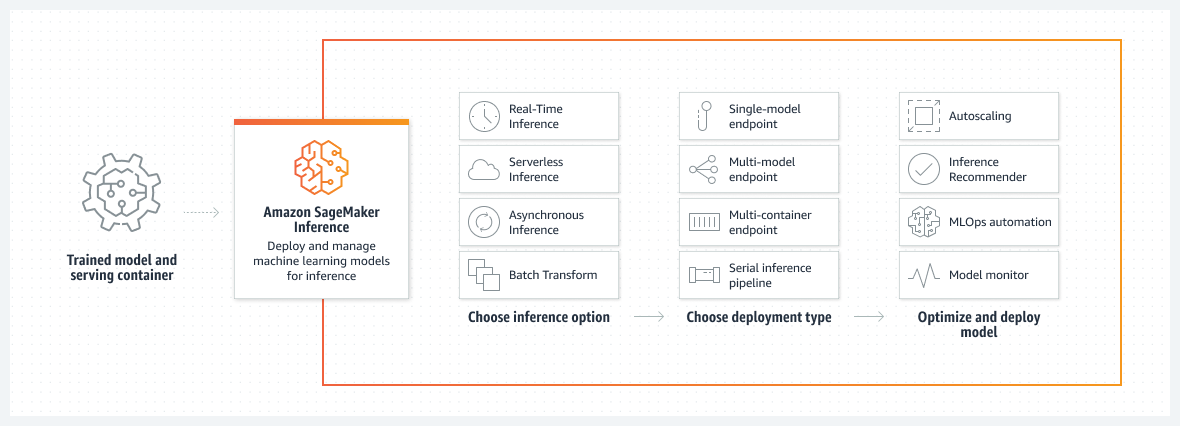
Wide range of options for every use case
Broad range of inference options
From low latency (a few milliseconds) and high throughput (hundreds of thousands of requests per second) to long-running inference for use cases such as natural language processing and computer vision, you can use Amazon SageMaker for all your inference needs.
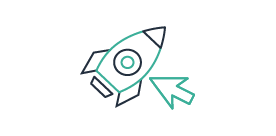
Real-Time Inference
Low latency and ultra-high throughput for use cases with steady traffic patterns.
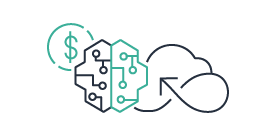
Serverless Inference
Low latency and high throughput for use cases with intermittent traffic patterns.
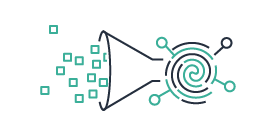
Asynchronous Inference
Low latency for use cases with large payloads (up to 1 GB) or long processing times (up to 15 minutes).
Flexible deployment endpoint options
Amazon SageMaker provides scalable and cost-effective ways to deploy large numbers of ML models. With SageMaker’s multi-model endpoints and multi-container endpoints, you can deploy thousands of models on a single endpoint, improving cost-effectiveness while providing the flexibility to use models as often as you need them. Multi-model endpoints support both CPU and GPU instance types, allowing you to reduce inference cost by up to 90%.
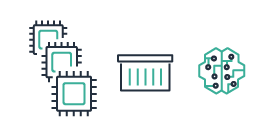
Single-model endpoints
One model on a container hosted on dedicated instances or serverless for low latency and high throughput.
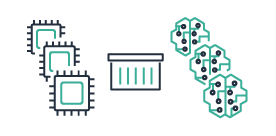
Multi-model endpoints
Multiple models sharing a single container hosted on dedicated instances for cost-effectiveness.
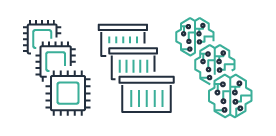
Multi-container endpoints
Multiple containers sharing dedicated instances for models that use different frameworks.
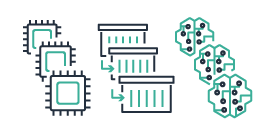
Serial inference pipelines
Multiple containers sharing dedicated instances and executing in a sequence.
Supports most machine learning frameworks and model servers
Amazon SageMaker inference supports built-in algorithms and prebuilt Docker images for some of the most common machine learning frameworks such as Apache MXNet, TensorFlow, and PyTorch. Or you can bring your own containers. SageMaker inference also supports most popular model servers such as TensorFlow Serving, TorchServe, NVIDIA Triton, and AWS Multi Model Server. With these options, you can deploy models quickly for virtually any use case.
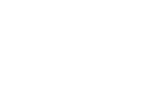
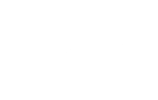
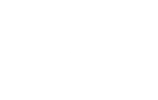
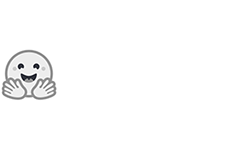
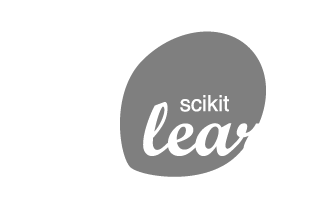
Achieve high inference performance at low cost
Deploy models on the most high-performing infrastructure or go serverless
Amazon SageMaker offers more than 70 instance types with varying levels of compute and memory, including Amazon EC2 Inf1 instances based on AWS Inferentia, high-performance ML inference chips designed and built by AWS, and GPU instances such as Amazon EC2 G4dn. Or, choose Amazon SageMaker Serverless Inference to easily scale to thousands of models per endpoint, millions of transactions per second (TPS) throughput, and sub10 millisecond overhead latencies.
Shadow test to validate performance of ML models
SageMaker helps you evaluate a new model by shadow testing its performance against the currently deployed model using live inference requests. Shadow testing can help you catch potential configuration errors and performance issues before they impact end users. With SageMaker, you don’t need to invest weeks of time building your own shadow testing infrastructure, meaning you can release models to production faster. Just select a production model that you want to test against, and SageMaker automatically deploys the new model in shadow mode and routes a copy of the inference requests received by the production model to the new model in real time. It then creates a live dashboard that shows performance metrics, such as latency and error rate, of the new model and the production model in a side-by-side comparison. Once you have reviewed the test results and validated the model, you can promote it to production.
Automatic inference instance selection and load testing
Amazon SageMaker Inference Recommender helps you choose the best available compute instance and configuration to deploy machine learning models for optimal inference performance and cost. SageMaker Inference Recommender automatically selects the compute instance type, instance count, container parameters, and model optimizations for inference to maximize performance and minimize cost.
Autoscaling for elasticity
You can use scaling policies to automatically scale the underlying compute resources to accommodate fluctuations in inference requests. With autoscaling, you can shut down instances when there is no usage to prevent idle capacity and reduce inference cost.
Reduce operational burden and accelerate time to value
Fully managed model hosting and management
As a fully managed service, Amazon SageMaker takes care of setting up and managing instances, software version compatibilities, and patching versions. It also provides built-in metrics and logs for endpoints that you can use to monitor and receive alerts.
Built-in integration with MLOps features
Amazon SageMaker model deployment features are natively integrated with MLOps capabilities, including SageMaker Pipelines (workflow automation and orchestration), SageMaker Projects (CI/CD for ML), SageMaker Feature Store (feature management), SageMaker Model Registry (model and artifact catalog to track lineage and support automated approval workflows), SageMaker Clarify (bias detection), and SageMaker Model Monitor (model and concept drift detection). As a result, whether you deploy one model or tens of thousands, SageMaker helps off-load the operational overhead of deploying, scaling, and managing ML models while getting them to production faster.
Customer success
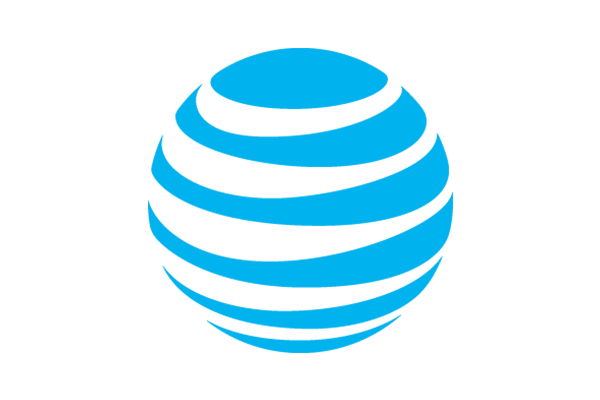
AT&T Cybersecurity improved threat detection requiring near-real-time predictions using Amazon SageMaker multi-model endpoints. “Amazon SageMaker multi-model endpoints are not only cost effective, but they also give us a nice little performance boost from simplification of how we store our models,”.
Matthew Schneid Chief Architect, AT&T
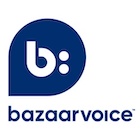
Bazaarvoice reduced ML inference costs by 82% using SageMaker Serverless Inference. “By using SageMaker Serverless Inference, we can do ML efficiently at scale, quickly getting out a lot of models at a reasonable cost and with low operational overhead.”
Lou Kratz, Principal Research Engineer – Bazaarvoice
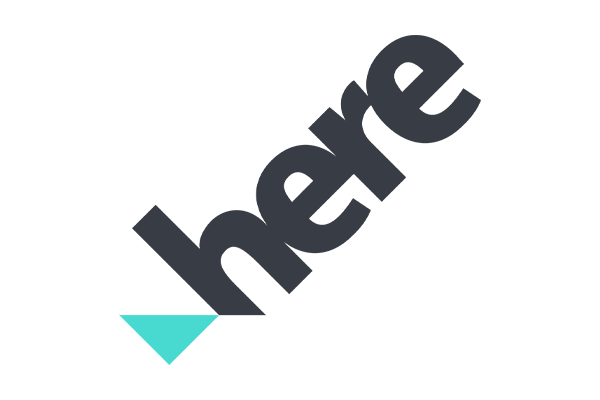
"Amazon SageMaker’s new testing capabilities allowed us to more rigorously and proactively test ML models in production and avoid adverse customer impact and any potential outages because of an error in deployed models. This is critical, since our customers rely on us to provide timely insights based on real-time location data that changes every minute.”
Giovanni Lanfranchi, chief product and technology officer – HERE Technologies
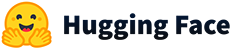
"Transformers have changed machine learning, and Hugging Face has been driving their adoption across companies, starting with natural language processing, and now, with audio and computer vision. The new frontier for machine learning teams across the world is to deploy large and powerful models in a cost-effective manner. We tested Amazon SageMaker Serverless Inference and were able to significantly reduce costs for intermittent traffic workloads, while abstracting the infrastructure. We’ve enabled Hugging Face models to work out-of-the-box with SageMaker Serverless Inference, helping customers reduce their machine learning costs even further."
Jeff Boudier, Director of Product – Hugging Face
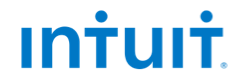
“With Amazon SageMaker, we can accelerate our Artificial Intelligence initiatives at scale by building and deploying our algorithms on the platform. We will create novel large-scale machine learning and AI algorithms and deploy them on this platform to solve complex problems that can power prosperity for our customers.”
Ashok Srivastava, Chief Data Officer – Intuit
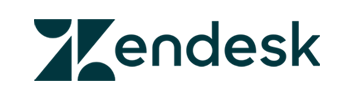
“We deployed thousands of ML models, customized for our 100K+ customers, using Amazon SageMaker multi-model endpoints (MME). With SageMaker MME, we built a multi-tenant, SaaS friendly inference capability to host multiple models per endpoint, reducing inference cost by 90% compared to dedicated endpoints.”
Chris Hausler, Head of AI/ML – Zendesk
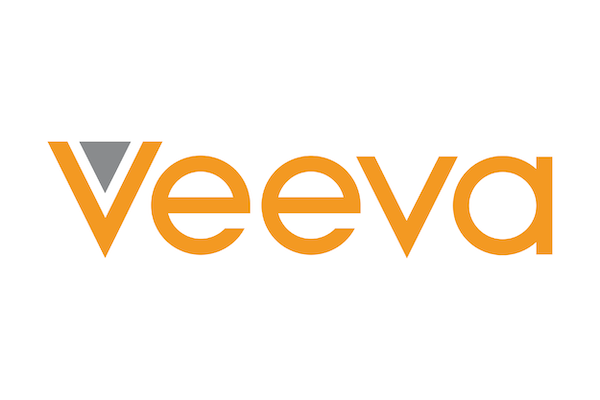
With Amazon SageMaker multi-model endpoints, Veeva scaled from ten models to hundreds of models using a much smaller number of real-time inference instances. That resulted in a reduction of operational costs due to the lower hosting cost and simpler endpoint management.
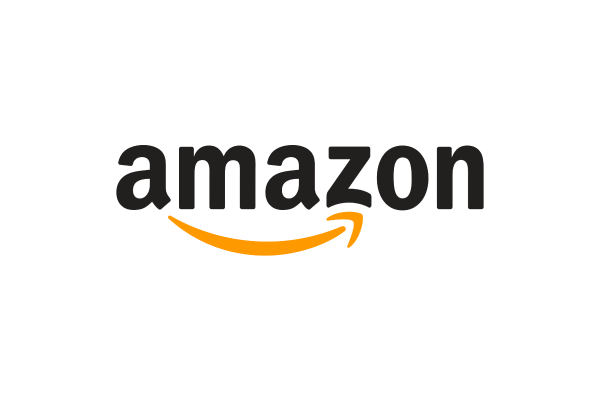
Amazon SageMaker freed the Amazon Robotics team from the difficult task of standing up and managing a fleet of GPUs for running inferences at scale across multiple regions. As of January 2021, the solution saved the company nearly 50 percent on ML inferencing costs and unlocked a 20 percent improvement in productivity with comparable overall savings. Continuing to optimize, at the end of 2021 the Robotics team shifted its deployment from GPU instances to AWS Inferentia-based Amazon EC2 Inf1 instances to save an additional 35 percent and see 20 percent higher throughput.
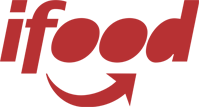
“iFood, a leading player in online food delivery in Latin America fulfilling over 60 million orders each month, uses machine learning to make restaurant recommendations to its customers ordering online. We have been using Amazon SageMaker for our machine learning models to build high-quality applications throughout our business. With Amazon SageMaker Serverless Inference we expect to be able to deploy even faster and scale models without having to worry about selecting instances or keeping the endpoint active when there is no traffic. With this, we also expect to see a cost reduction to run these services.”
Ivan Lima, Director of Machine Learning & Data Engineering – iFood
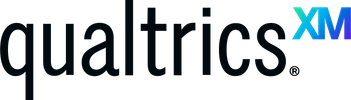
“Amazon SageMaker Inference Recommender improves the efficiency of our MLOps teams with the tools required to test and deploy machine learning models at scale. With SageMaker Inference Recommender, our team can define latency and throughput requirements and quickly deploy these models faster, while also meeting our budget and production criteria.”
Samir Joshi, ML Engineer – Qualtrics
Resources
AWS re:Invent 2022 - Deploy ML models for inference at high performance & low cost, ft AT&T (AIM302)
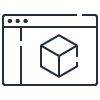
Follow this step-by-step tutorial to deploy a model for inference using Amazon SageMaker.
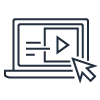
In this hands-on lab, learn how to use Amazon SageMaker to build, train, and deploy an ML model.
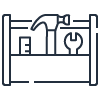
Get started building with Amazon SageMaker in the AWS Management Console.